Design Massively Scalable Unique ID Generator
Get started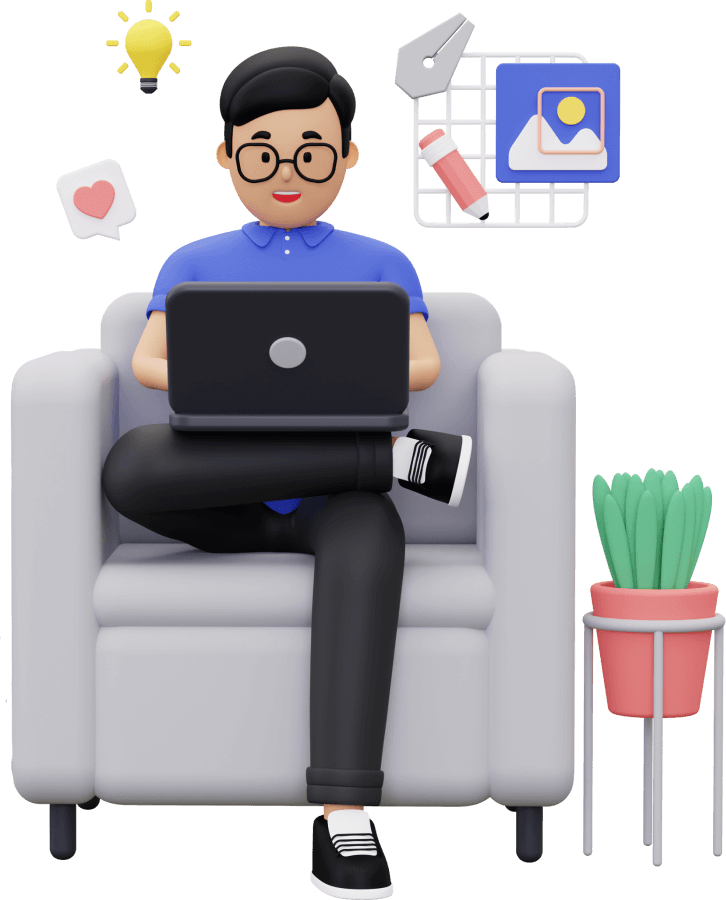
জয় শ্রী রাম
🕉General advice for System Design Interview:
-
Be a Consultant – imagine your interviewer is a customer you’ll be building something for, consider a tangible system, from requirements to post-production, and what that means.
- Consider the customer – is this a good experience? What purpose does it serve?
-
Drive the conversation by asking great questions and validating assumptions.
-
Know Why – Defending design tradeoffs is critical.
-
Interesting Discussion – show advanced or unique edge cases – validate your assumptions and
ask the right questions.
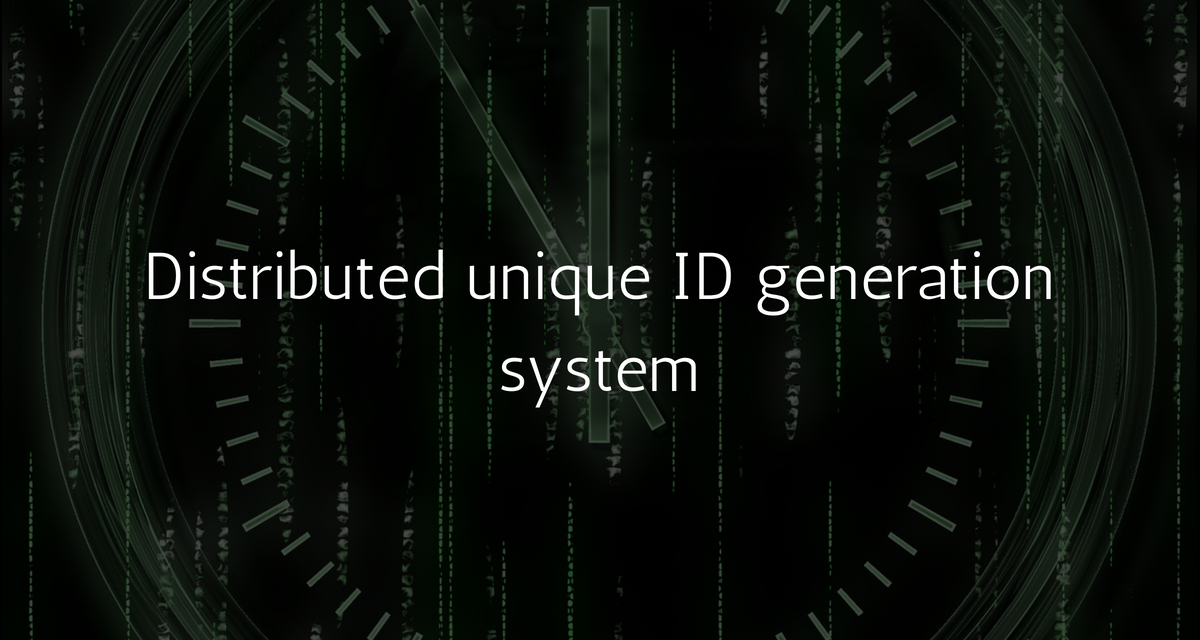
Login to Access Content